AWS S3 is a versatile product, used for many different purposes from logs to big data storages. It’s basic operations are easy to implement using the AWS SDK.
For the first step we need to create an S3 bucket which will hold our files. Go to AWS console, search for S3 product in the search bar:
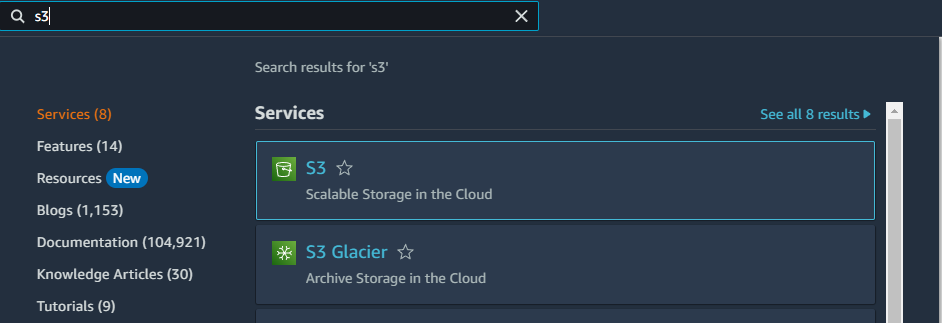
Click on Create bucket button:

Choose a name and region, then hit Create bucket at the bottom of the page:
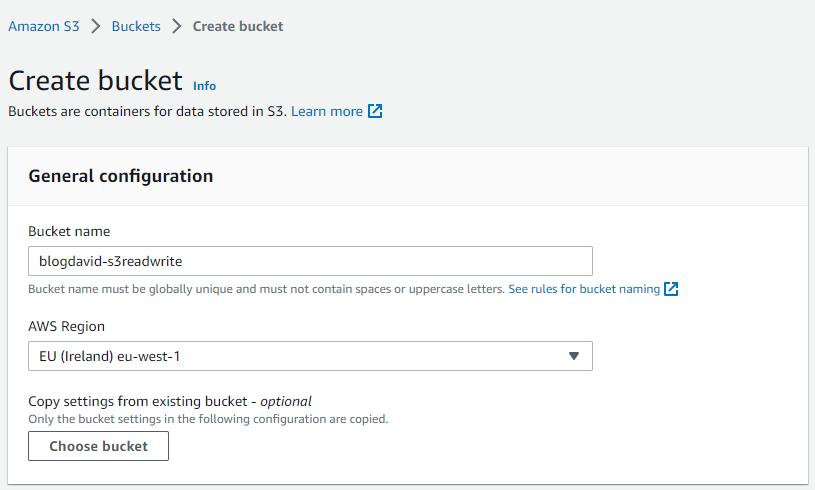
Now we are all set on the AWS console, we are going to use it later on for testing.
We can get back to our code editor and add AWSSDK.S3 to our project, which has bunch of S3 related codes to help us out. Let’s write some code:
using Amazon.S3;
using Amazon.S3.Model;
using System.Text;
namespace BlogDavid_S3ReadWrite
{
internal class Program
{
// Note: Running the program will use the local machine's credentials!
// Store the bucket's name somewhere
const string s3BucketName = "blogdavid-s3readwrite";
// Set the client to our bucket's region
static IAmazonS3 s3Client = new AmazonS3Client(Amazon.RegionEndpoint.EUWest1);
static async Task Main(string[] args)
{
// Generating a random path for our text file
string randomizedPath = $"{Guid.NewGuid()}/{Guid.NewGuid()}/{Guid.NewGuid()}.txt";
// Adding some text to save
string textToSave = $"I am a text generated at {DateTime.UtcNow}";
// Converting the text into bytes
byte[] textAsBytes = Encoding.UTF8.GetBytes(textToSave);
using (MemoryStream ms = new MemoryStream(textAsBytes))
{
// Rewinding the stream
ms.Position = 0;
PutObjectRequest putRequest = new PutObjectRequest
{
// Name of the bucket
BucketName = s3BucketName,
// Key as the path under the bucket
Key = randomizedPath,
// Data we are saving
InputStream = ms,
};
await s3Client.PutObjectAsync(putRequest);
}
// Let's read back the previous file
// Construction the request object
GetObjectRequest getRequest = new GetObjectRequest
{
BucketName = s3BucketName,
Key = randomizedPath
};
// Sening the request
using (var getResponse = await s3Client.GetObjectAsync(getRequest))
using (var sr = new StreamReader(getResponse.ResponseStream))
{
// Reading back the response
var getResponseString = await sr.ReadToEndAsync();
// Showing the response, which is hopefully the text we just saved
Console.WriteLine(getResponseString);
}
Console.ReadKey();
}
}
}
Starting our app, it should write and read a file on S3:

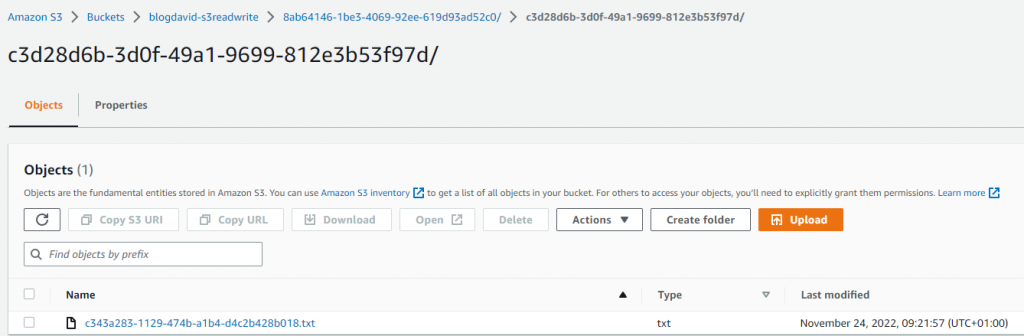
Happy coding!